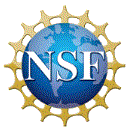
Modeling and Analysis of Green Mobile Crowd Sensing
Sponsored by the U.S. National Science Foundation
Welcome to the website of our research project: “Modeling and Analysis of Green Mobile Crowd Sensing”. This website is created and maintained to disseminate and share research results and other information related to the project.
Project Description
Mobile crowd sensing (MCS) arises as a new sensing paradigm based on the power of the crowd together with the ever-increasing sensing capabilities of various mobile devices. As carrying out sensing tasks can deplete the energy of battery-powered mobile devices quickly, this concern largely affects the wide deployment of MCS. Therefore, how to enhance the energy efficiency in MCS is an imperative and challenging task. Inspired by recent advances in wireless networking and energy harvesting techniques, the proposed research aims to develop joint sensing task computation and communication framework to achieve green MCS for various sensing tasks. The research project and activities have significant potential to better support newly emerging MCS applications such as healthcare, environment monitoring, traffic monitoring, social behavior monitoring, etc. The research results are expected to inspire other theoretical and systematic studies to contribute to the networking design and energy management aspects of developing energy-efficient MCS. The project plans to engage female and under-represented minority students in the research activities. The results of the project will be disseminated through publications and talks.
This project has an exciting two-year research plan focusing on fundamental challenges associated with modeling and analyzing green MCS. Observing that the energy consumed in task processing and its distribution correlates to each other, a unified framework to jointly model the energy consumption in computation and communication is proposed to strike a balance between the two to achieve energy efficiency. As renewable energy has emerged as a feasible alternative to the traditional energy sources, it is incorporated in the sensing crowd so as to decrease the on-grid energy demand from sensing devices. Dynamic energy optimization problems are then investigated to minimize energy expenditure in supporting performance-guaranteed sensing tasks, by comprehensively considering time-varying computing resource allocation, renewable energy supply, and wireless channel conditions. Moreover, some sensing devices are envisioned to be capable of transferring extra harvested renewable energy to others nearby, so as to fully explore the vacant energy and computation resources in MCS. Finally, in order to stimulate mobile devices to join MCS, incentive mechanisms and heterogeneous auction markets are developed.
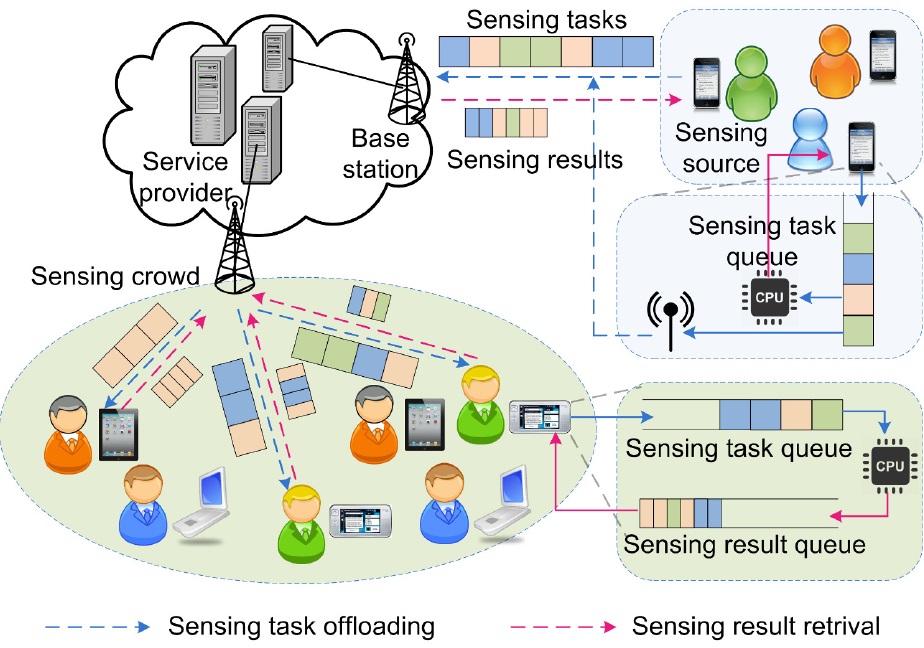
Publications
-
STEP: A Spatio-Temporal Fine-Granular User Traffic Prediction System for Cellular Networks ,
Lixing Yu, Ming Li, Wenqiang Jin, Yifan Guo, Qianlong Wang, Feng Yan, and Pan Li,
IEEE Transactions on Mobile Computing (TMC), 2020.
-
Data-Driven Spectrum Trading with Secondary Users' Differential Privacy Preservation ,
Jingyi Wang, Qixun Zhang, Ming Li, Yuanxiong Guo, Zhiyong Feng, and Miao Pan,
IEEE Transactions on Dependable and Secure Computing (TDSC), 2019.
-
If You Do Not Care About It, Sell It: Trading Location Privacy in Mobile Crowd Sensing ,
Wenqiang Jin, Mingyan Xiao, Ming Li, and Linke Guo,
Proceedings of IEEE International Conference on Computer Communications (INFOCOM'19).
-
Securing Task Allocation in Mobile Crowd Sensing: An Incentive Design Approach,
Mingyan Xiao, Ming Li, Linke Guo, Miao Pan, Zhu Han and Pan Li,
Proceedings of IEEE Conference on Communications and Network Security (CNS'19).
-
Crowd-empowered privacy-preserving data aggregation for mobile crowdsensing
Lei Yang, Mengyuan Zhang, Shibo He, Ming Li, and Junshan Zhang,
ACM International Symposium on Mobile Ad Hoc Networking and Computing (MobiHoc'18).
-
Motivating Human-Enabled Mobile Participation for Data Offloading,
Xiaonan Zhang, Linke Guo, Ming Li, and Yuguang Fang,
IEEE Transactions on Mobile Computing (TMC), Vol. 17, No. 7, pp. 1624-1637, July 2018.
-
DPDA: A Differentially Private Double Auction Scheme for Mobile Crowd Sensing,
Wenqiang Jin, Ming Li, Linke Guo, and Lei Yang,
IEEE Conference on Communications and Network Security (CNS'18).
-
Economic-Robust Transmission Opportunity Auction for D2D Communications in Cognitive Mesh Assisted Cellular Networks,
Ming Li, Weixian Liao, Xuhui Chen, Jinyuan Sun, Xiaoxia Huang, and Pan Li,
IEEE Transactions on Mobile Computing (TMC), pp. 1-1, December 2017.
-
Pricing, Caching Selection, and Content Delivery in Wireless Networks: A Hierarchical Approach,
Heli Zhang, Bowen Liu, Ming Li, Hong Ji, Xi Li, and Victor Leung,
IEEE Global Communications Conference (GLOBECOM'17).
-
Energy-efficient Autonomic Offloading in Mobile Edge Computing,
Changqing Luo, Sergio Salinas, Ming Li, and Pan Li,
IEEE Digital Avionics Systems Conference (DASC'17).
-
Energy-source-aware cost optimization for green cellular networks with strong stability,
Weixian Liao, Ming Li, Sergio Salinas, Pan Li, and Miao Pan,
IEEE Transactions on Emerging Topics in Computing (TETC), Vol. 4, No. 4, pp. 541-555, October 2016.
-
Social-enabled data offloading via mobile participation-a game-theoretical approach,
Xiaonan Zhang, Linke Guo, Ming Li, and Yuguang Fang,
IEEE Global Communication Conferences (GLOBECOM'16).
-
Privacy-Preserving Data Aggregation over Incomplete Data for Crowdsensing,
Iman Vakinilia, Jiajun Xin, Ming Li, and Linke Guo,
IEEE Global Communications Conference (GLOBECOM'16).
-
Privacy-preserving Verifiable Data Aggregation and Analysis for Cloud-assisted Mobile Crowdsourcing,
Gaoqiang Zhuo, Qi Jia, Linke Guo, Ming Li, and Pan Li,
IEEE International Conference on Computer Communications (INFOCOM'16).
-
Privacy-Preserving Verifiable Set Operation in Big Data for Cloud-assisted Mobile Crowdsourcing,
Gaoqiang Zhuo, Qi Jia, Linke Guo, Ming Li, and Pan Li,
IEEE Internet of Things Journal (IoT), Vol. 4, No. 2, pp. 572-582, June 2016.